Blog Post
I don't think I am revealing too much in saying that two years ago I had the honor of being the first crowd researcher to be funded by CloudQuant.
I have to admit it has been a struggle since, but I have eventually managed to set up a reasonable way of trading multi-day strategies.
I want to share where I think I went wrong: I put a focus on the risk allocation, rather than factors. And, if I'm honest, over-fit risk allocations. I've come to the conclusion that the focus should, instead, be on the factors, with a simple approach to risk allocation. Related to this, I also think the sophistication should be in the predictive models and not the risk allocation.
I've re-focused my goals towards enjoying the process.
In the following strategy, which runs right up through the recent turmoil, I managed to maintain a portfolio of 2,000 positions, exiting on the final day such that only 1 position was left open.
I'm going to try to get enjoyment by achieving milestones such as this, rather than dreaming about riches.
With the caveat that, at this stage I am just trying to get the strategies to run successfully to completion, the strategy runs for 2 years with a nice firework display suggesting it could be submitted for funding!
If my analysis is correct, this strategy is also close to being beta-neutral with respect to SPY.
After some more experiments, I am now confident about how to avoid the dreaded "Backtest Failed to Complete" message. I also like the economics, which appear to be positively-skewed returns and lower turnover.
Either way, I now have an initial base template, with which I can fine tune the risk management.
The next step I am working on is combining signals, and maintaining a constant dollar exposure of $10m. The simulation runs to completion with no problem for the 1-year period commencing in 2012.
I've been working on using a dynamic universe (specifically the S&P 1500). Successfully ran to completion for the last year, and coped reasonably well with all the recent turmoil:
And finally, a 3-year backtest runs to completion using a dynamic universe.
At this stage, the set-up is simple naive diversification, but at least the performance is consistent with the minimum FundMyStrategy conditions, even allowing for recent market conditions.
The next step is to improve the strategy using an appropriate risk allocation.
I do this by training on a 3-year window and then setting the risk allocation for the next year (not on the same time period, which unsurprisingly generates spectacular results!).
The data looks very reliable for the last 3 years, so any problems in the training set should be manageable, as I don't see a reason why they would cause a systematic bias.
Now the training window is rolled forward by a year to include the period 2016-19. The resulting factor combination is then run on the unseen period 2019-20.
The exciting news is that the full walk-forward simulation ran to completion and successfully generated a scorecard. Furthermore, because I had run each of the three years separately, I was able to validate the single simulation against the individual years by looking at the P/L on individual days.
I had an experiment with jointly making a portfolio dollar-neutral and zero-beta, and it's a bit of a non-starter. I'm quite happy with the framework of the strategy above, as it's simple and seems to always generate a scorecard.
An alternative, which I may design later, is to split the universe by size or beta in the first instance, running in effect two separate portfolios. This helps to mitigate beta risk, while maintaining dollar-neutrality.
Comments
I now have a related project in mind, where the goal is to use the available resources to construct a realistic walk-forward analysis.
I use 2012 as the warm-up period. Learning now occurs for the first time when rebalancing ahead of 2013.
2012-13, as a trader could have done it in real time. The portfolio is now rebalanced ahead of 2014.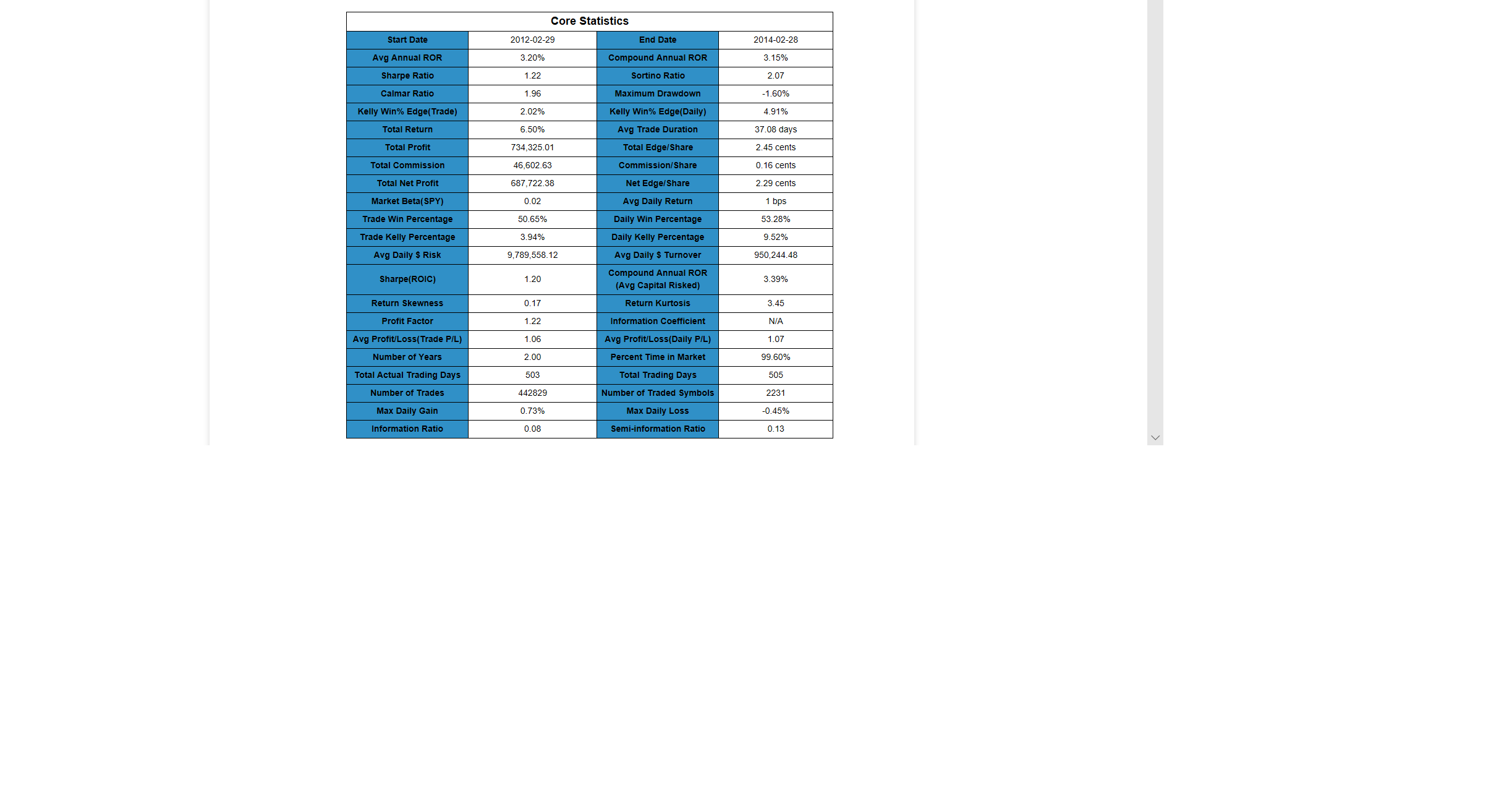
2012-14: 2014 was a difficult year for this famous vanilla factor!
2012-15:
2015 was a spectacularly good year for the factor, but I am expecting a large drawdown in 2016.
Annual rebalance ahead of 2016.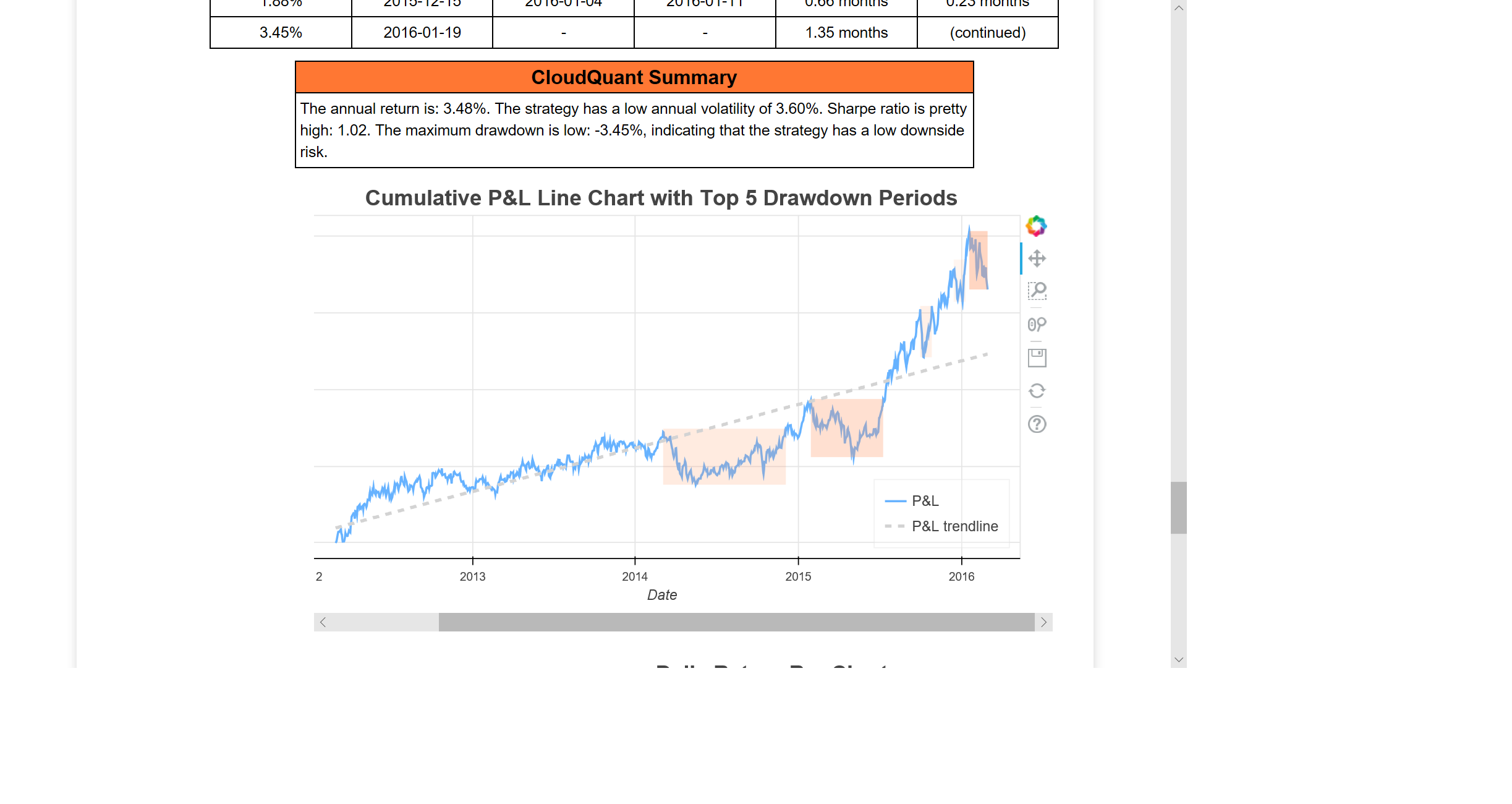
Zero-beta strategy with walk-forward testing...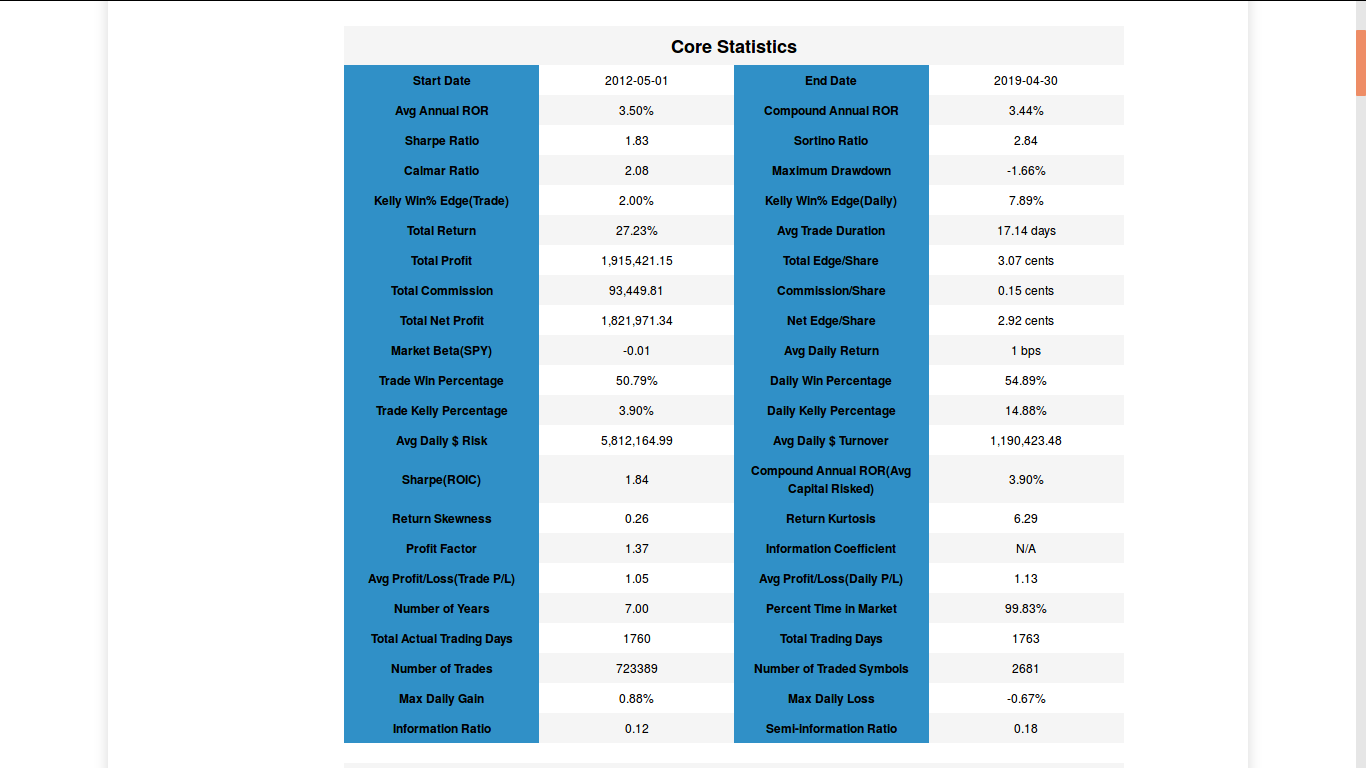
Blog Post
I don't think I am revealing too much in saying that two years ago I had the honor of being the first crowd researcher to be funded by CloudQuant.
I have to admit it has been a struggle since, but I have eventually managed to set up a reasonable way of trading multi-day strategies.
I want to share where I think I went wrong: I put a focus on the risk allocation, rather than factors. And, if I'm honest, over-fit risk allocations. I've come to the conclusion that the focus should, instead, be on the factors, with a simple approach to risk allocation. Related to this, I also think the sophistication should be in the predictive models and not the risk allocation.
I've re-focused my goals towards enjoying the process.
In the following strategy, which runs right up through the recent turmoil, I managed to maintain a portfolio of 2,000 positions, exiting on the final day such that only 1 position was left open.
I'm going to try to get enjoyment by achieving milestones such as this, rather than dreaming about riches.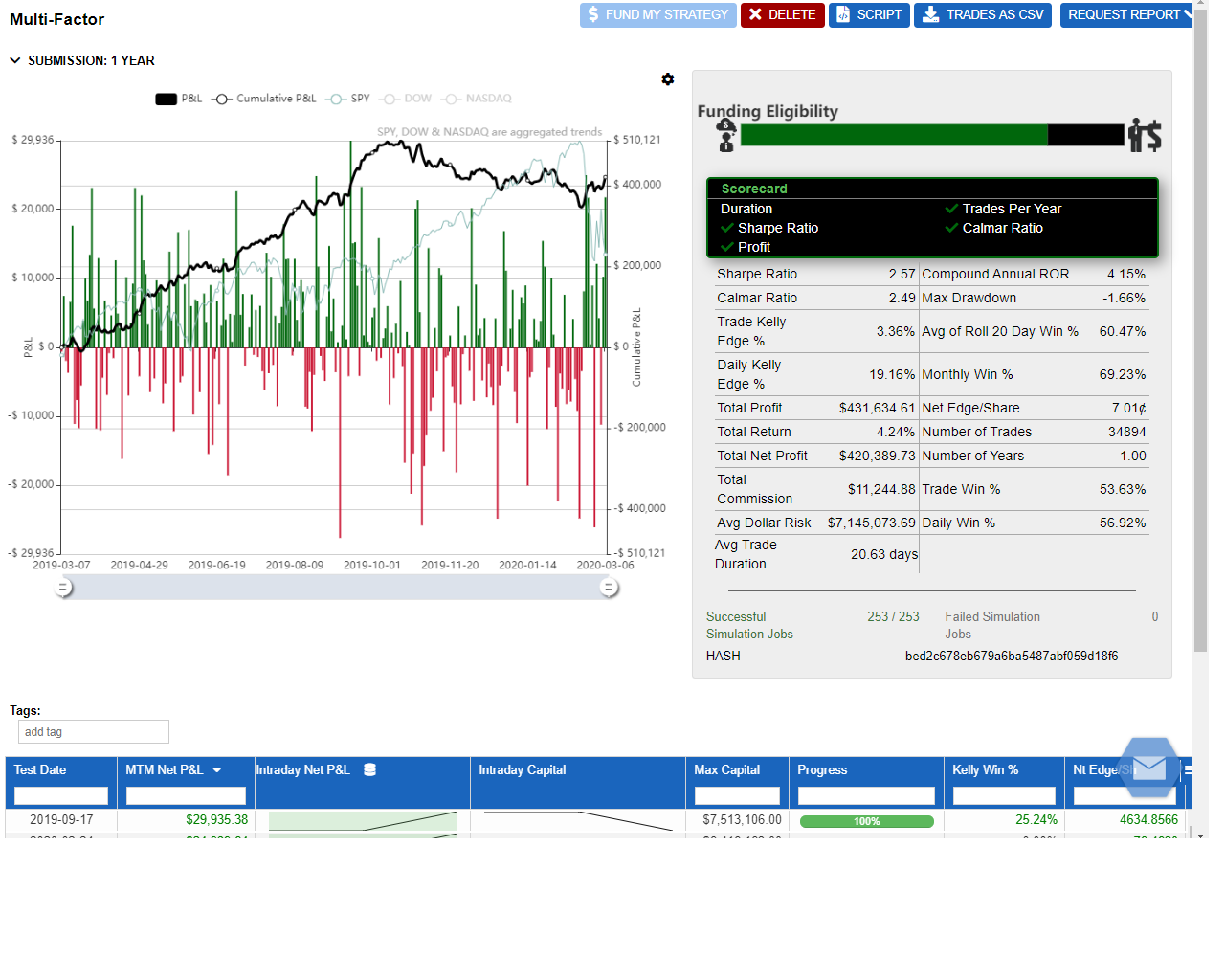
...and a screenshot showing the number of positions, with a full exit on Friday 6th March, 2020.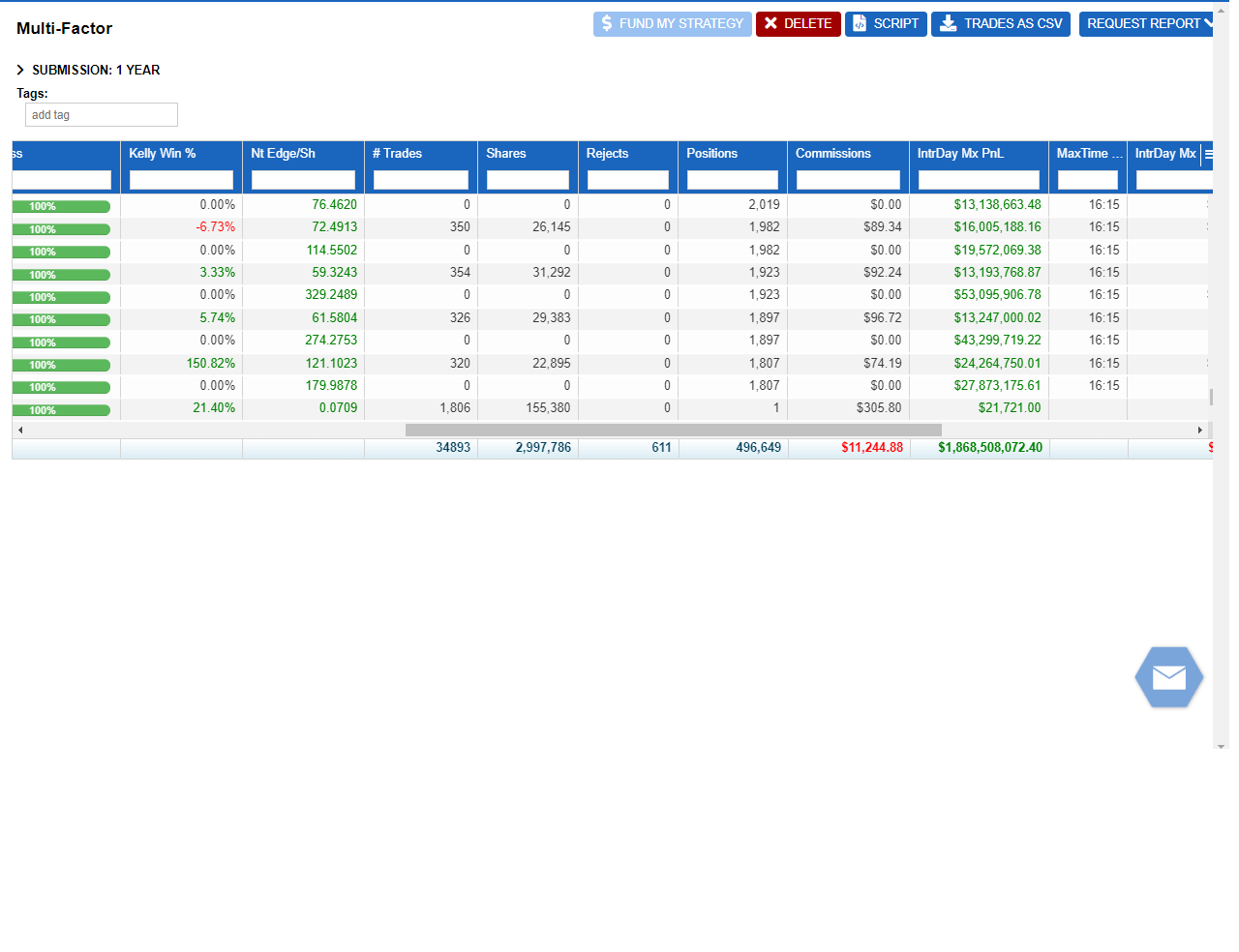
With the caveat that, at this stage I am just trying to get the strategies to run successfully to completion, the strategy runs for 2 years with a nice firework display suggesting it could be submitted for funding!
If my analysis is correct, this strategy is also close to being beta-neutral with respect to SPY.
Ideally, I would like to be able to run a 3 or 4 year simulation, with the scorecard working, and work with a long backtest in two parts.
At this stage, the main positive is that 2 years can be run without apparently introducing a systematic bias.
After some more experiments, I am now confident about how to avoid the dreaded "Backtest Failed to Complete" message. I also like the economics, which appear to be positively-skewed returns and lower turnover.
Either way, I now have an initial base template, with which I can fine tune the risk management.
The next step I am working on is combining signals, and maintaining a constant dollar exposure of $10m. The simulation runs to completion with no problem for the 1-year period commencing in 2012.
The SPY beta for the simulation is -0.03
I've been working on using a dynamic universe (specifically the S&P 1500). Successfully ran to completion for the last year, and coped reasonably well with all the recent turmoil: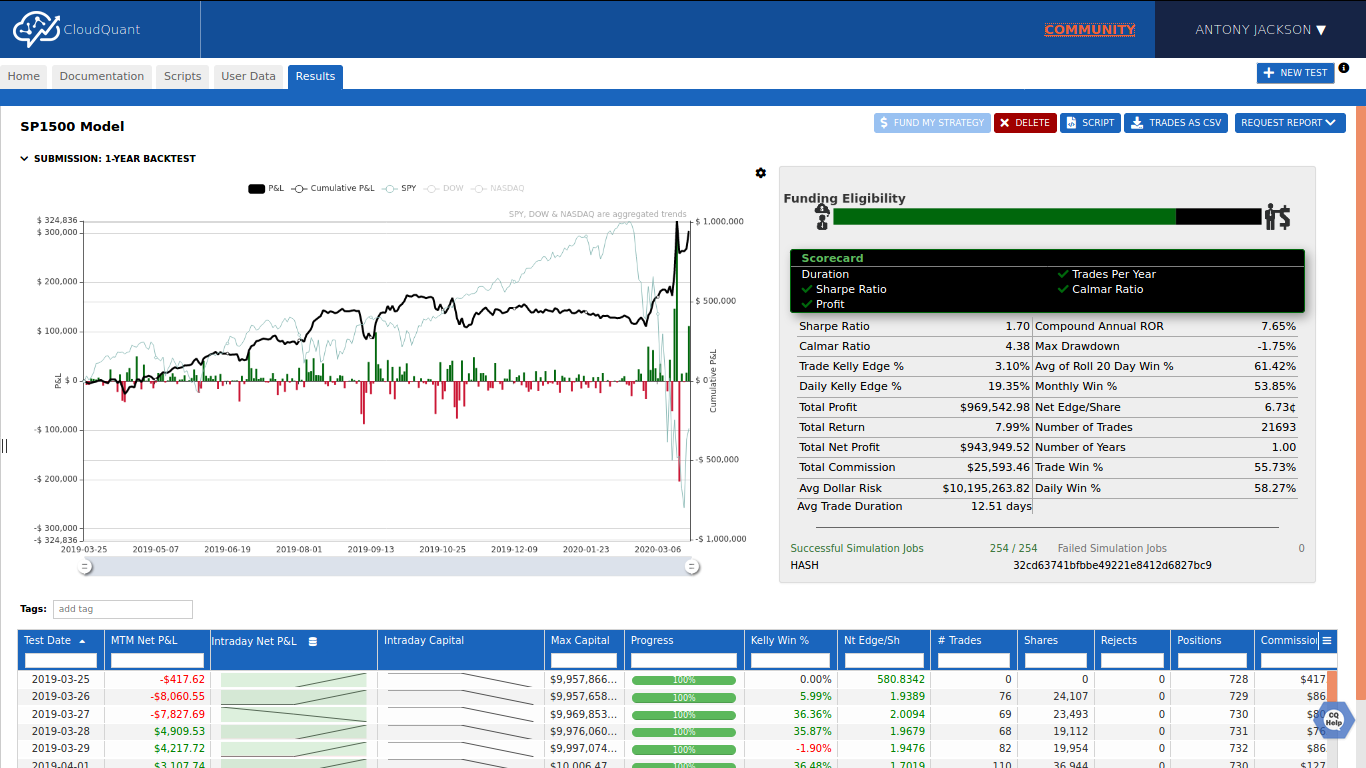
Also works for a 2-yea backtest, with the FundMyStrategy button lighting up...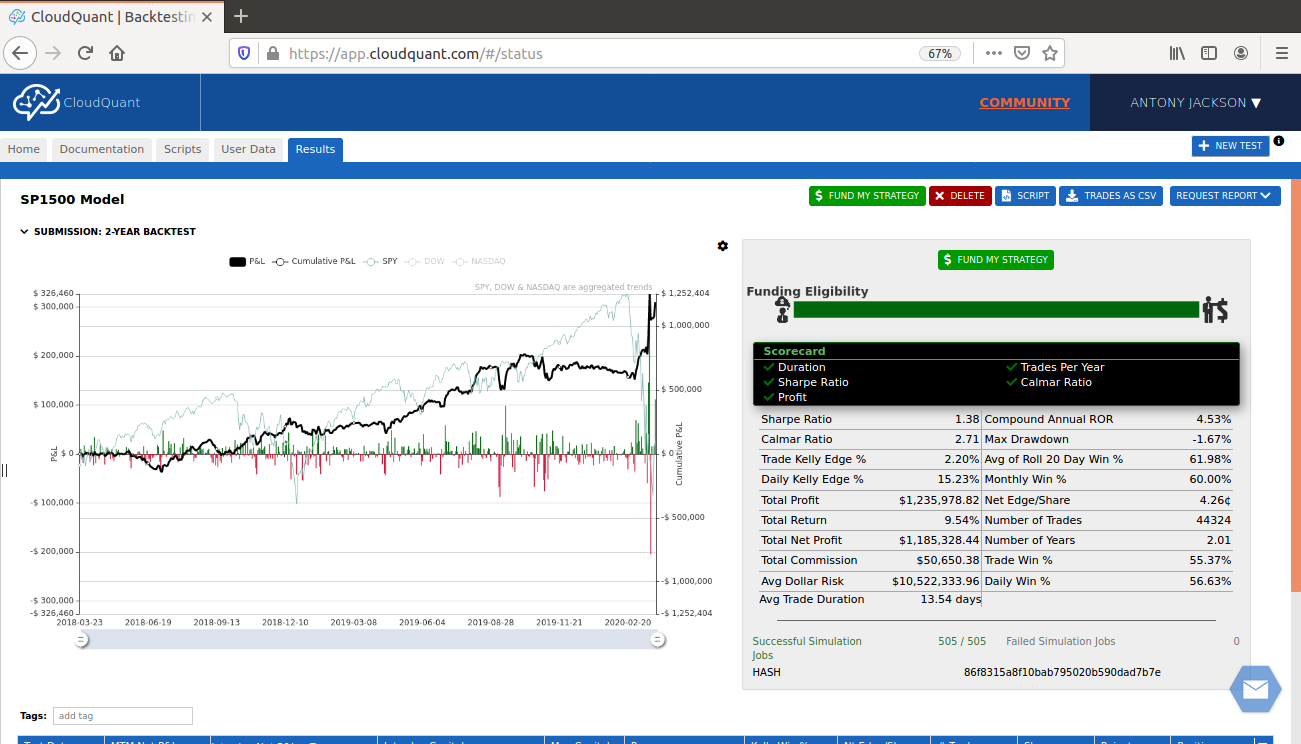
And finally, a 3-year backtest runs to completion using a dynamic universe.
At this stage, the set-up is simple naive diversification, but at least the performance is consistent with the minimum FundMyStrategy conditions, even allowing for recent market conditions.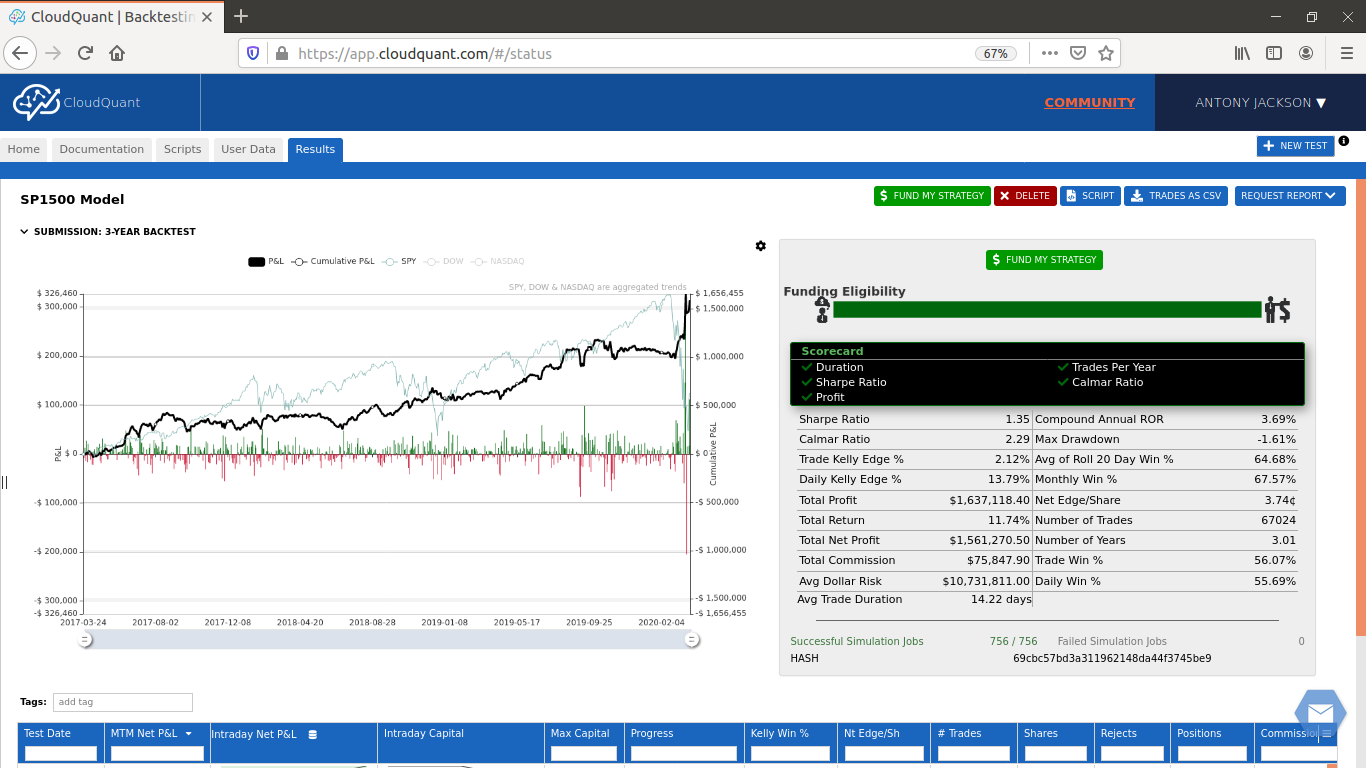
The next step is to improve the strategy using an appropriate risk allocation.
I do this by training on a 3-year window and then setting the risk allocation for the next year (not on the same time period, which unsurprisingly generates spectacular results!).
The data looks very reliable for the last 3 years, so any problems in the training set should be manageable, as I don't see a reason why they would cause a systematic bias.
Walk-forward analysis next.
After using the 2014-17 period to determine risk allocations for 2017-18, I obtained the following results.
The next step is to roll forward the 3-year training period by one year in order to determine the risk allocations for the 2018-19 period.
Here are the results for the walk forward period 2018-19:
Now the training window is rolled forward by a year to include the period 2016-19. The resulting factor combination is then run on the unseen period 2019-20.
Here are the results for the walk forward period 2019-20:
Finally, I will try to combined these all into one algorithm that updates the risk allocation annually.
The exciting news is that the full walk-forward simulation ran to completion and successfully generated a scorecard. Furthermore, because I had run each of the three years separately, I was able to validate the single simulation against the individual years by looking at the P/L on individual days.
Here are the results:
To summarize the work this week:
I started using a dynamic universe based on the S&P 1500, with error-checking in case of gaps in the lists.
I used walk-forward analysis to generate dynamic risk allocations (without looking into the future).
The strategy satisfies the conditions for applying for funding, so I will take it from there.
I had an experiment with jointly making a portfolio dollar-neutral and zero-beta, and it's a bit of a non-starter. I'm quite happy with the framework of the strategy above, as it's simple and seems to always generate a scorecard.
An alternative, which I may design later, is to split the universe by size or beta in the first instance, running in effect two separate portfolios. This helps to mitigate beta risk, while maintaining dollar-neutrality.
That concludes the journal.